Image-based quality control “Damage Detection”
Automated inspection of damage and defects using AI-assisted quality control of reflective surfaces.
Where does the AI application offer the greatest benefit?
The inspection of reflective surfaces, for example of quality defects in production or damage to vehicles, is often still carried out manually and therefore turns out to be time-consuming and requires a high level of expertise on the part of the persons in charge. In order to increase the productivity of the processes, “Damage Detection”, an AI system for the quality control of shiny or diffusely reflecting surfaces, has been developed. It is suitable for a wide range of applications: from industrial production, for example in the automotive industry, to the leasing and insurance industry and to automotive appraisals. The system works automatically and takes less than a minute for surface inspection. The quality defects found are categorized using Deep Learning. The combination of deflectometry, i.e. the contact-free detection of reflective surfaces, conventional image recognition processes and artificial intelligence methods is a unique system.
What are the quality indicators of such AI applications?
“The combination of conventional image recognition
methods, AI methods and the detection of reflective
surfaces is unique.”
What AI technology powers the demonstrator of KI.NRW?
The solution relies on Convolutional Neural Networks (CNNs) for detection and classification of the surface anomalies. The CNN model belongs to the Artificial Neural Networks, which are suitable for image recognition and understanding.
By incorporating expert knowledge, the system is able to make dataintensive neural networks practical for industrial production. The amount of training data and the annotation effort can be kept comparatively low.
The AI is linked to classical image processing. The system uses the advantages of both worlds – fast, approximate algorithms from classical image processing and the powerful methods of deep learning.
What does the AI demonstrator show?
Quality control of shiny or diffusely reflecting surfaces is notable for its simple, mobile hardware design. It operates independently of ambient light and is fully automated. It takes less than one minute for a surface inspection.
Where is more information to be found?
Image recognition in practice
Many “good practice examples” as inspiration for the possible applications of this AI.
AI products “made in NRW”
Filter our AI map by “image recognition and understanding”.
AI provider from NRW
Our AI map illustrates who offers AI methods related to image recognition in their portfolio.
Contact us
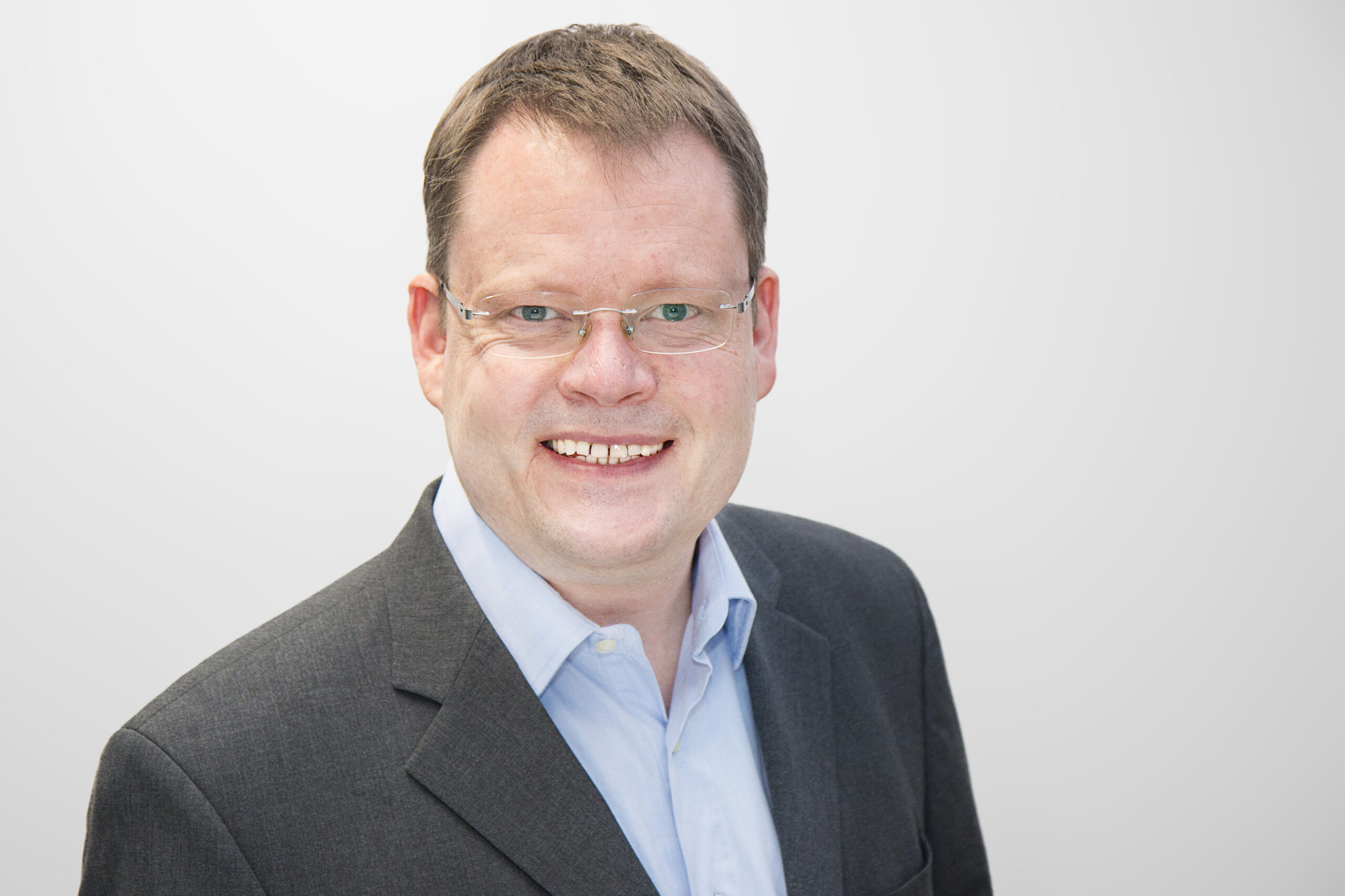
Dr.-Ing. Stefan Eickeler
Senior Research Engineer
Fraunhofer IAIS
Schloss Birlinghoven
53757 Sankt Augustin
Phone +49 2241 141969